
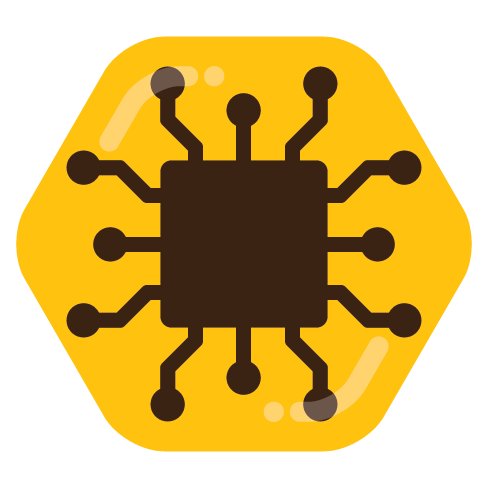
deleted by creator
deleted by creator
I think this is downplaying what LLMs do. Yeah, they are not the best at doing things in general, but the fact that they were able to learn the structure and semantic context of language is quite impressive, even if it doesn’t know what the words converted into tokens actually mean. I suspect that we will be able to use LLMs as one part of a full digital “brain”, with some model similar to our own prefrontal cortex calling the LLM (and other things like vision model, sound model, etc.) and using its output to reason about a certain task and take an action. That’s where I think the hype will be validated, is when you put all these parts we’ve been working on together and Frankenstein a new and actually intelligent system.
For the love of God please stop posting the same story about AI model collapse. This paper has been out since May, been discussed multiple times, and the scenario it presents is highly unrealistic.
Training on the whole internet is known to produce shit model output, requiring humans to produce their own high quality datasets to feed to these models to yield high quality results. That is why we have techniques like fine-tuning, LoRAs and RLHF as well as countless datasets to feed to models.
Yes, if a model for some reason was trained on the internet for several iterations, it would collapse and produce garbage. But the current frontier approach for datasets is for LLMs (e.g. GPT4) to produce high quality datasets and for new LLMs to train on that. This has been shown to work with Phi-1 (really good at writing Python code, trained on high quality textbook level content and GPT3.5) and Orca/OpenOrca (GPT-3.5 level model trained on millions of examples from GPT4 and GPT-3.5). Additionally, GPT4 has itself likely been trained on synthetic data and future iterations will train on more and more.
Notably, by selecting a narrow range of outputs, instead of the whole range, we are able to avoid model collapse and in fact produce even better outputs.
We have no moat and neither does OpenAI is the leaked document you’re talking about
It’s a pretty interesting read. Time will tell if it’s right, but given the speed of advancements that can be stacked on top of each other that I’m seeing in the open source community, I think it could be right. If open source figured out scalable distributed training I think it’s Joever for AI companies.
I don’t know what type of chatbots these companies are using, but I’ve literally never had a good experience with them and it doesn’t make sense considering how advanced even something like OpenOrca 13B is (GPT-3.5 level) which can run on a single graphics card in some company server room. Most of the ones I’ve talked to are from some random AI startup that have cookie cutter preprogrammed text responses that feel less like LLMs and more like a flow chart and a rudimentary classifier to select an appropriate response. We have LLMs that can do the more complex human tasks of figuring out problems and suggesting solutions and that can query a company database to respond correctly, but we don’t use them.
Blocking out the sun with aerosols is a good idea if you know with high confidence how it will impact the climate system and environment. That’s why they’re trying to simulate it with the supercomputer, so they know if it fucks stuff up or not.
This makes sense for any other company but OpenAI is still technically a non profit in control of the OpenAI corporation, the part that is actually a business and can raise capital. Considering Altman claims literal trillions in wealth would be generated by future GPT versions, I don’t think OpenAI the non profit would ever sell the company part for a measly few billions.
Lmao Twitter is not that hard to create. Literally look at the Mastodon code base and “transform” it and you’re already most of the way there.
The natural next place for people to go to once they can’t block ads on YouTube’s website is to go to services that exploit the API to serve free content (NewPipe, Invidious, youtube-dl, etc.). If that happens at a large scale, YouTube might shut off its API just like Reddit did and we’ll end up in scenario where creators are forced to move to Peertube, and, given how costly hosting is for video streaming, it could be much worse than Reddit->Lemmy+KBin or Twitter->Mastodon. Then again, YouTube has survived enshittiffication for a long time, so we’ll have to wait and see.
FediSearch I guess is similar to your idea, though I think the goal would be to make a new and open search index specifically containing fediverse websites instead of just using Google. I also feel like the formatting should be more like Lemmy, with the particular post title and short description showing instead of the generic search UI.
The idea of a fediverse search is really cool though. If things like news and academic papers ever got their own fediverse-connected service, I could see a FediSearch being a great alternative to the AI sludge of Google.
Basically he is pro-privacy, somewhere in the libertarian space, supports usage of monero, recommends you move to a rural area, etc.
I can’t think of a time he’s said any slur, but there is a particular video I would be interested to see it
I definitely agree. The vast majority of people still left on Reddit are those who are corporate bootlickers and those who do not care and just want to doom scroll.
Neither type adds anything to an online community
This isn’t an actual problem. Can you train on post-ChatGPT internet text? No, but you can train on the pre-ChatGPT common crawls, the millions of conversations people have with the models and on audio, video and images. As we improve training techniques and model architectures, we will need even less of this data to train even more performant models.
The one SIMPLE trick crypto bros HATE: Blockchain -> “Distributed Ledger” NFT -> “Unique Identifier”
Like and share with your friends
There are some in the research community that agree with your take: THE CURSE OF RECURSION: TRAINING ON GENERATED DATA MAKES MODELS FORGET
Basically the long and short of that paper is that LLMs are inherently biased towards likely responses. The more their training set is LLM generated, and thus contains that bias, the less the LLM will be able to produce unlikely responses, over time degrading the model quality throughout successive generations.
However, I tend to think this viewpoint is probably missing something important. Can you train a new LLM on today’s internet? Probably not, at least without some heavy cleaning. Can you train a multimodal model on video, audio, the chat logs of people talking to it, and even other better LLMs? Yes, and you will get a much higher quality model and likely won’t get the same model collapse implied by the paper.
This is more or less what OpenAI has done. All the conversations with 100M+ users are saved and used to further train the AI. Their latest GPT4 is also trained on video and image recognition, and they have also been exploring ways for LLMs to train new ones, especially to aid in alignment of these models.
Another recent example is Orca, a fine tune of the open source llama model, which is trained by GPT-3.5 and GPT-4 as teachers, and retains ~90% of GPT-3.5’s performance though it uses a factor of 10 less parameters.
NFTs are stupid AF for most of the tasks people currently use them for and definitely shouldn’t be used as proof of ownership of physical assets.
However, I think NFTs make a lot of sense as proof of ownership of purely digital assets, especially those which are scarce.
For example, there are several projects for domain name resolution based on NFT ownership (e.g you look up crypto.eth, your browser checks that the site is signed by the owner of the crypto.eth NFT, then you are connected to the site), as it could replace our current system, which has literally 7 guys that hold a private key that is the backbone of the DNS system and a bunch of registrars you have to go through to get a domain. This won’t happen anytime soon but it is an interesting concept.
Then I think an NFT would also be good as a decentralized alternative to something like Google sign in, where you sign up for something with the NFT and sign in by proving your ownership of it.
In general though I find NFTs to be a precarious concept. I mean the experience I’ve had with crypto is you literally have a seed phrase for your wallet, and if it gets stolen all your funds are drained. And then for an NFT, if you click on the wrong smart contract, all your monkeys could be gone in an instant. There is in general no legal recourse to reverse crypto transactions, and I think that is frankly the biggest issue with the technology as it stands today.