
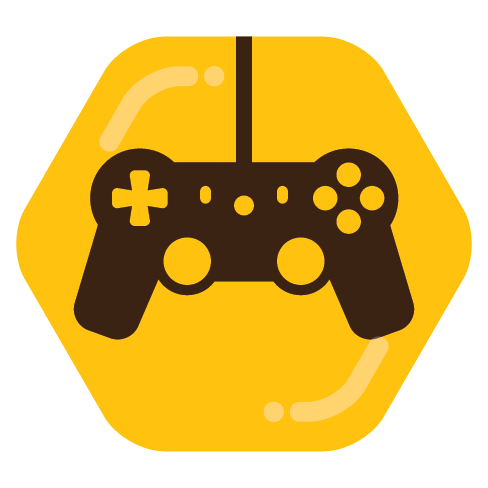
Any good options recommended for self-hosting something similarly functional that doesn’t take too much effort to get up, audit, maintain? Discovery isn’t really important for me, so federated isn’t really necessary, but a cool extra. I’d love to host something or contribute to hosting for my gaming groups, my class or multiple classes at my school, or otherwise. Voice, chat, screen share, camera, would all be great if possible, but range of options would be good. I’m still using Mumble for gaming…
Haven’t tinkered much with Matrix nor do I know much about Revolt, but I’m curious before I look into it deeper if anyone in the community has experience hosting any communication platforms for small, invitational groups.
Can’t say I’m deep in this space, but I think there’s a lot of sentiment towards going more lean with operations and aiming for direct donation toward Firefox development (which I don’t believe is presently an option) which seemingly, if Mozilla narrowed to their core (Firefox, MDN), the community would likely show heavy support. I have my doubts it would fully cover the bill in a sustainable way, but I at least think that’s one of the main sentiments.